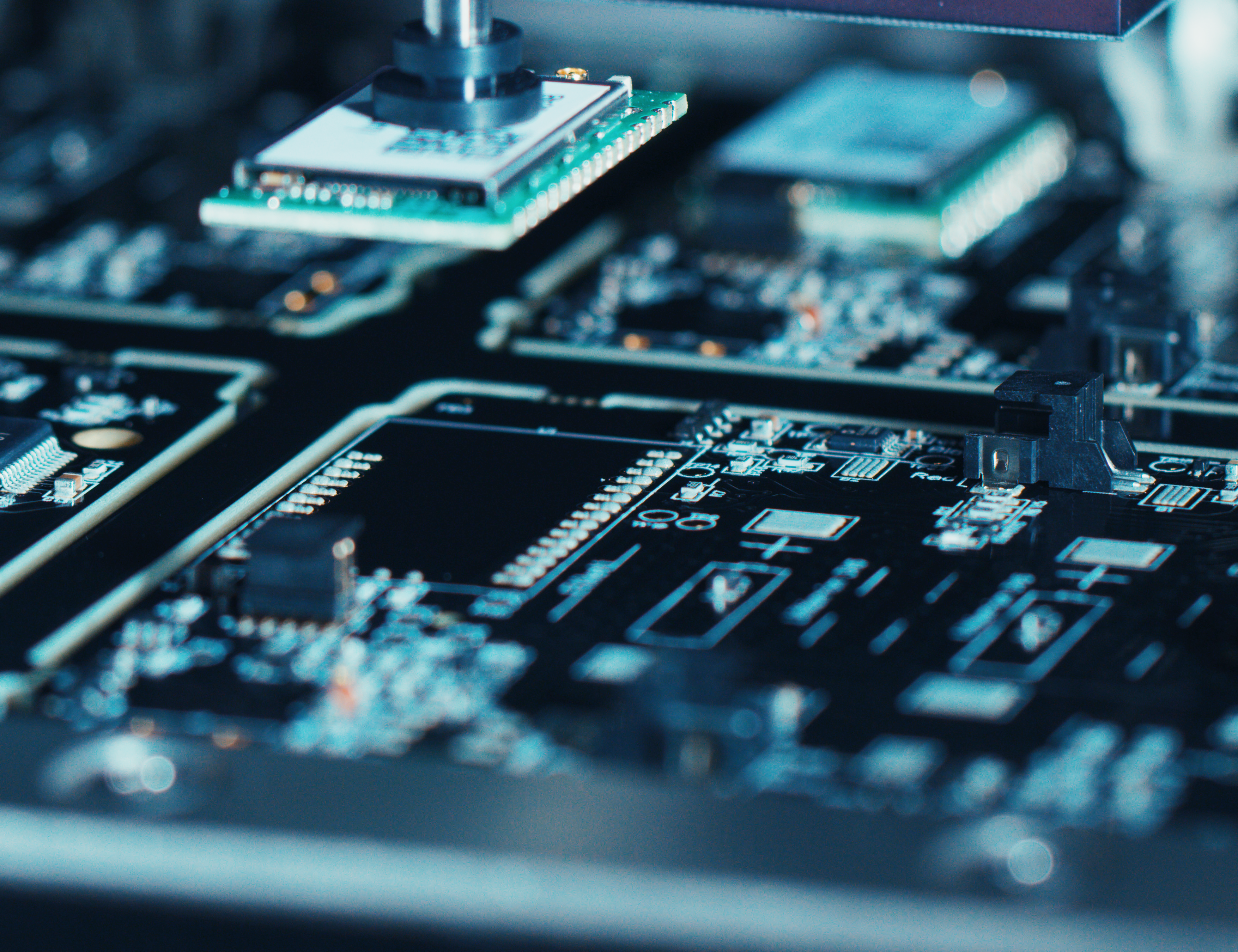
- Manufacturing
- Optimization
SMT Performance: Optimized in 8 Weeks
Leverage reinforcement learning to optimize electronic component mounting sequences on PCBs, reducing overall process time in SMT.
READ MOREIn the manufacturing sector, software-driven motion control solutions are pivotal for managing various machines and fixtures. However, a major issue arises when the equipment and facility parameters are not finely tuned. This results in discrepancies between the control values set by the motion control software and the actual values executed by the equipment driver. Traditional manual tuning, carried out by on-site engineers, often varies in quality and efficiency due to differing skill levels, leading to inconsistent tuning results. This inconsistency hampers the establishment of a uniform technical standard across the enterprise.
This strategy led to a remarkable 52% reduction in the time required for tuning, compared to traditional expert-led methods. Moreover, we observed a significant improvement in precision, with up to a 20% decrease in discrepancies between the control values commanded by the motion control system and the actual outputs from the machine driver. Our AI-driven solution represents a groundbreaking shift in the automation of parameter tuning, delivering rapid and accurate adjustments irrespective of the operator's skill level.
Compared to expert-driven tuning, we achieved an impressive reduction in tuning time by up to 52%, while simultaneously increasing accuracy by up to 20%. This enhancement ensures a much closer alignment between the intended commands and the actual behavior of the machines.
Want to learn more about this use case?
Get in touch with our industrial AI experts.